PROJECTS
Natural Language Generation
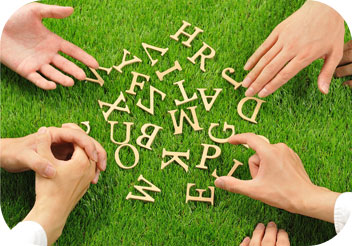
Our research is aimed at establishing a generic framework to automatically produce natural language descriptions of numerical data such as stock market statistics. This framework will be an essential component for an automated system to comprehend real-world activities, which we can observe as numerical data.
Applications of our framework include:
- Automatic generation of market summaries
- Automatic generation of weather conditions
- Live broadcasting
Vision and Language
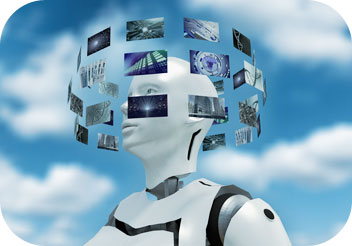
We are combining computer vision, natural language processing, and machine learning to enable computers to understand images/videos, rather than just working at pixel levels. Currently we are working on automatic video description generation, which can be useful in many aspects. Generated descriptions enable computers to understand images/videos, because they contain objects appearing in the videos along with their attributes, locations, actions, and relations with other objects. With the generated video descriptions, we can automatically summarize and query the contents of videos, and it also can help visually-impaired people to understand videos better and easier.
In addition to automatic video description generation, we also have plans for other tasks such as:
- Video entity linking
- Image/video-based question answering
- Video summarization
- Image/video querying
NLP for Finance
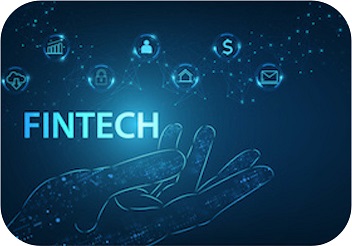
Our goal is to develop an AI professional analyst to alleviate the asymmetric information problem in the financial market. That is, we attempt to improve information transparency in the financial market with natural language processing (NLP) and artificial intelligence (AI) methods. We believe that the results of the proposed research directions will improve fairness in the financial market.
Our research mainly focuses on:
- Financial Opinion Mining
- Forward-Looking Argument Mining
- Professional Report Generation
- Causality and Rationale Inference
- Finance for Social Good
- Numeracy of PLM
- Financial Technology (FinTech)
- Regulation Technology (RegTech)